This term, a proprietary system, likely represents a complex model or platform. Its specific function and components are not publicly documented. Without further context, it's challenging to provide a concrete definition. Potentially, it encompasses a variety of interconnected data streams, algorithms, or a specific approach to a particular field, such as global market analysis, resource management, or data modeling. Its exact application requires external information.
The value of such a system hinges on its specific capabilities. If it provides a novel approach to processing, interpreting, and leveraging data within a given field, it could offer significant advantages, such as enhanced predictive modeling, improved decision-making, or optimization of resource allocation. However, its actual impact depends on the concrete functionalities and successful implementation. Further investigation into the system's capabilities and how it is used in practice would be necessary to fully assess its potential benefits.
This article will now explore the potential uses of such systems within the field of [mention a specific area, e.g., international finance]. We will examine how these kinds of platforms can potentially enhance [mention a specific aspect, e.g., risk assessment].
cinas_worldx
Understanding the key aspects of "cinas_worldx" is crucial for evaluating its potential applications. Its multifaceted nature demands a comprehensive approach.
- Data Integration
- Model Complexity
- Predictive Capability
- Scalability
- Algorithmic Design
- Real-World Application
- Resource Allocation
The seven aspects collectively paint a picture of "cinas_worldx" as a sophisticated, potentially transformative system. Data integration underscores its ability to process vast datasets, while model complexity suggests advanced predictive capabilities. Scalability implies wide-reaching applications, and algorithmic design highlights the technical ingenuity behind it. Real-world application demonstrates its utility, and resource allocation suggests optimizational potential. The potential to leverage "cinas_worldx" for improved prediction and resource efficiency across industries suggests an innovative platform, possibly with significant impact in areas like market analysis or logistics.
1. Data Integration
Data integration is a foundational component of any complex system, and its role within "cinas_worldx" is likely pivotal. Effective data integration ensures that disparate data sources are unified and accessible, enabling comprehensive analysis and informed decision-making. The success of "cinas_worldx" hinges on its ability to seamlessly combine diverse information streams, from market trends to real-time sensor data, to create a cohesive and actionable dataset.
- Data Source Heterogeneity
The system's effectiveness depends on its ability to reconcile various data formats and structures, such as structured databases, unstructured text, and streaming feeds. Successfully merging these often-incompatible sources is critical for generating a comprehensive overview. Failure to standardize and align these diverse inputs will result in inaccurate or incomplete analyses.
- Real-Time Data Incorporation
Integration with real-time data streams, such as financial markets or sensor readings, is likely crucial. This capacity for dynamic data updates is essential to inform near-term actions and facilitate real-time adjustments to models or strategies. Examples include incorporating live stock prices for market analysis or sensor data for resource allocation.
- Data Cleansing and Validation
Data integration necessitates procedures for cleaning, validating, and transforming raw data. This preprocessing is essential for removing inconsistencies, errors, and redundancies. The integrity and reliability of the analyzed data depend directly on these processes. Examples include handling missing values, standardizing units, and identifying erroneous entries.
- Security and Privacy Considerations
Data security and privacy protocols are paramount within the context of "cinas_worldx." Data integration must be performed adhering to regulatory standards and ethical guidelines to protect sensitive information. Failure to address these aspects could expose the system and its users to significant risks.
In essence, the quality of data integration directly impacts the accuracy, reliability, and applicability of insights derived from "cinas_worldx." A robust data integration framework is a prerequisite for leveraging the potential of the system, allowing it to deliver accurate predictions and actionable intelligence across various applications.
2. Model Complexity
The level of model complexity inherent in "cinas_worldx" is critical to its potential effectiveness. Sophisticated models are necessary to capture the intricate relationships within the datasets the system processes. The degree of complexity directly influences the accuracy and reliability of predictions and insights derived from the system.
- Variable Interactions
The model's ability to account for intricate interdependencies between variables is crucial. Accurate predictions require the model to understand how changes in one factor affect others. Real-world examples include macroeconomic models that link interest rates, inflation, and employment or supply chain models tracking the impact of disruptions across multiple components. Within "cinas_worldx," this capacity for recognizing complex interactions will determine the system's ability to anticipate emergent behavior and make nuanced predictions.
- Non-linear Relationships
Many real-world phenomena exhibit non-linear patterns. A simple linear model often fails to capture these dynamics. The accuracy of "cinas_worldx" depends on its ability to accommodate non-linear relationships, ensuring it doesn't oversimplify complex interactions. For instance, logistic growth models, which represent population or market expansion, demonstrate how non-linearity is essential for precise representation. Models in "cinas_worldx" needing to understand such relationships will necessitate sophisticated algorithms and potentially more complex architectures.
- Data Dimensionality
The volume and variety of data processed by "cinas_worldx" likely involve high dimensionality. This means models must effectively handle multiple variables. Techniques like dimensionality reduction or feature selection are crucial to manage computational complexity and ensure accurate predictions. For example, models employed in financial analysis routinely work with many market variables. "cinas_worldx," facing similar challenges, must be equipped with methods to efficiently manage this high dimensionality without losing critical information.
- Robustness to Noise and Outliers
Real-world data often contains errors, inaccuracies, or outliers. A robust model in "cinas_worldx" must effectively filter this noise and not be unduly influenced by these anomalous data points. This characteristic is essential for creating reliable predictions in dynamic environments. Examples include models for detecting fraudulent transactions or predicting equipment failures, both requiring a high level of resilience against unpredictable data deviations.
Ultimately, the appropriate level of model complexity in "cinas_worldx" hinges on striking a balance between capturing the nuanced interactions within the data and maintaining computational feasibility. Higher complexity can potentially lead to greater predictive power, but it also increases the risk of overfitting to the training data, compromising the model's generalization capabilities.
3. Predictive Capability
The predictive capability of a system like "cinas_worldx" is paramount to its value. Its ability to forecast future trends, anticipate potential events, or model complex scenarios directly impacts the efficacy of its applications. Accurate predictions are foundational to informed decision-making in diverse fields, from resource allocation to risk management.
- Forecasting Trends and Patterns
The system's predictive capabilities are likely built upon algorithms designed to identify and extrapolate trends from historical data. Success depends on the model's ability to recognize subtle patterns and predict how these patterns will evolve over time. Real-world applications include stock market forecasting, weather prediction, and even sales forecasting for businesses. Within "cinas_worldx," accurate trend recognition is essential to anticipate future market shifts, resource demands, or potential disruptions.
- Anticipating Events and Impacts
Beyond simple trend identification, the system's sophistication may extend to anticipating events and evaluating their potential consequences. Examples include models anticipating supply chain disruptions or predicting the impact of policy changes on various sectors. For "cinas_worldx," this capability would allow for proactive adjustments and mitigation of potential risks or capitalizing on favorable opportunities.
- Complex Scenario Modeling
The system may employ complex models to simulate future outcomes under various hypothetical conditions. This capacity is valuable for scenario planning, risk assessment, and strategy development. Examples include evaluating the potential consequences of different energy policies or modeling the effects of a global pandemic on a specific industry. Applying this capability to "cinas_worldx" would enable strategic decisions regarding future investments, diversification, or other proactive responses.
- Model Refinement and Calibration
Predictive capabilities are not static. The system's predictive accuracy relies on ongoing refinement and calibration. Models must be adjusted based on new data, evolving conditions, and performance metrics. Continuous validation and improvement are crucial for maintaining the accuracy and reliability of predictions over time. Such adaptation is essential for "cinas_worldx" to remain relevant and accurate as external conditions shift and new data become available.
The predictive capabilities of "cinas_worldx" directly influence its usefulness. The system's success depends on its ability to offer reliable insights to support informed decision-making. Failure to deliver accurate predictions would diminish the overall value of the platform.
4. Scalability
Scalability, in the context of a system like "cinas_worldx," refers to its ability to adapt and expand its processing capacity to handle growing datasets, increasing user demands, and evolving analytical needs. A scalable system can effectively manage an expanding volume of data without significant performance degradation. This characteristic is crucial for long-term viability and sustained value. Without scalability, the system's effectiveness might diminish as the scope of its application grows. Examples include online retail platforms: initially designed for a limited number of users and products, they must scale to accommodate explosive growth in both customer base and inventory.
The practical importance of scalability within "cinas_worldx" lies in its potential to serve a broad range of applications and users. Consider an initial deployment for a specific industry sector. Scalability allows the platform to expand to other sectors or geographical locations. Suppose "cinas_worldx" is designed for optimizing supply chains; initial usage within one company could potentially expand to encompass an entire industry by virtue of its scalability. This ability to accommodate diverse applications and expand user base ensures the platform remains relevant over time. A non-scalable system will likely encounter performance issues or become unusable as its load increases, potentially hindering the system from reaching its full potential.
Ultimately, scalability is not merely a desirable attribute but a foundational requirement for a system like "cinas_worldx" to realize its full potential. The ability to adapt to changing demands is crucial for sustaining value and utility over an extended period. Success hinges on the system's capacity to accommodate future growth in data volume and user interactions. Challenges in scalability often include maintaining efficiency and accuracy as the system grows larger, but these are usually surmountable with appropriate design considerations. A thoughtfully designed scalable system ensures long-term viability and successful integration into various applications and markets.
5. Algorithmic Design
The effectiveness of a system like "cinas_worldx" hinges critically on its algorithmic design. Algorithms form the core logic governing data processing, analysis, and prediction. Sophisticated algorithms are essential for handling the multifaceted nature of the data involved, enabling the system to identify complex patterns, model intricate relationships, and generate accurate predictions. Without robust algorithms, the system would be limited in its ability to extract meaningful insights. Consider a financial trading algorithm: its efficacy depends entirely on the design and implementation of the underlying trading logic, including variables like order placement, risk assessment, and profit maximization strategies. Analogously, "cinas_worldx" requires carefully crafted algorithms to process, interpret, and synthesize vast quantities of diverse data.
The intricate design of algorithms within "cinas_worldx" influences several crucial aspects. Data integration, for instance, depends heavily on algorithms that harmonize diverse data formats and sources. The system's predictive capability stems from algorithms that learn patterns from historical data and extrapolate them to forecast future outcomes. Furthermore, the scalability of "cinas_worldx" directly correlates with the efficiency and adaptability of its algorithms. Efficient algorithms are vital for handling substantial datasets and user demands without significant performance degradation. Examples of sophisticated algorithmic design in financial modeling, natural language processing, and image recognition demonstrate how optimized algorithms are crucial for complex systems.
Understanding the algorithmic design of "cinas_worldx" provides valuable insights into the system's underlying architecture and functionalities. This understanding facilitates a critical evaluation of its strengths and weaknesses. The design directly impacts the system's accuracy, efficiency, and ability to adapt to various applications and evolving data inputs. Challenges in algorithmic design, such as overfitting to training data or computational complexity, can diminish the system's overall performance and utility. Further exploration of the specific algorithms used in "cinas_worldx" will provide a clearer perspective on its potential and limitations. Careful consideration of the algorithmic design is fundamental to assessing the system's long-term viability and potential impact.
6. Real-World Application
The practical application of a system like "cinas_worldx" is crucial for evaluating its true potential. Effective implementation within real-world scenarios demonstrates the system's value and utility. This section explores key facets of real-world application, examining its components and significance in relation to "cinas_worldx." Understanding these facets provides insights into the system's capabilities and limitations.
- Industry-Specific Deployment
Successful application often involves tailoring the system to particular industries. This customized approach ensures relevance and efficacy. For instance, if "cinas_worldx" is intended for financial markets, specialized algorithms for risk assessment and portfolio optimization are essential. Adapting the system to the intricacies of different industries whether healthcare, energy, or agriculture is a critical factor in successful implementation. The effectiveness of "cinas_worldx" is measured by its relevance and impact within these industry-specific deployments.
- Integration with Existing Infrastructure
Seamless integration with existing business processes and technological infrastructure is essential for practical application. A system that requires extensive restructuring or custom development might not be economically viable or efficient. The integration process must be considered when evaluating the feasibility and practicality of deploying "cinas_worldx." Difficulties in this integration could limit its adoption. Assessing the compatibility between the system's architecture and existing tools is paramount for practical deployment.
- Performance Metrics and Evaluation
Defining and monitoring key performance indicators (KPIs) is critical to evaluating the system's success in real-world applications. These KPIs should reflect the specific goals of each application, whether it's improving operational efficiency, forecasting market trends, or enhancing decision-making. Without measurable performance metrics, the system's effectiveness remains difficult to assess. Comprehensive evaluations should incorporate analysis of predictive accuracy, resource utilization, and integration with existing processes. This detailed assessment provides crucial feedback for system improvement and refinement.
- Data Privacy and Security Protocols
Practical application mandates compliance with stringent data privacy and security regulations. The system must protect sensitive data and comply with relevant laws and regulations. This facet underscores the importance of secure data handling. Compliance with these guidelines is essential for responsible and ethical deployment. Robust security protocols and adherence to privacy standards are necessary for successful and sustainable implementation of "cinas_worldx," preventing potential misuse or breaches.
Ultimately, the real-world application of "cinas_worldx" demands careful consideration of industry context, technological integration, performance measurement, and data security. Successful deployment hinges on these critical components. The ability to adapt to various industries and integrate seamlessly into existing infrastructure will directly influence the system's acceptance and overall value. Critically evaluating how well "cinas_worldx" performs these aspects will significantly impact its adoption rate and overall usefulness.
7. Resource Allocation
Resource allocation, a core component of efficient operations, becomes particularly relevant when considering a system like "cinas_worldx." The system's potential lies in its capacity to optimize resource allocation across various domains. Understanding the intricacies of this application is crucial for assessing "cinas_worldx"'s overall value.
- Optimizing Utilization
Efficient resource allocation hinges on optimizing utilization. "cinas_worldx" could leverage predictive modeling to forecast future needs, enabling proactive allocation strategies. This anticipatory approach could avoid bottlenecks and ensure resources are deployed where and when they're most needed. For example, in manufacturing, predicting equipment maintenance needs based on usage patterns could optimize resource allocation for preventative maintenance, minimizing downtime and maximizing production efficiency. This directly impacts "cinas_worldx"'s potential to offer cost-effective strategies and improve efficiency in various sectors.
- Prioritizing Needs
"cinas_worldx" may offer the capability to prioritize competing demands for resources. Data-driven analysis, facilitated by the system, could aid in assessing the urgency and importance of different requests. This prioritization could be vital in dynamic environments where resource constraints are common. For instance, in healthcare, the system could aid in allocating medical resources, such as personnel or equipment, based on patient acuity and urgency, thereby improving patient outcomes. This prioritization function is a crucial element for a system like "cinas_worldx" in complex operational scenarios.
- Predictive Modeling and Forecasting
The system might integrate predictive modeling to forecast resource needs. By analyzing historical data and current trends, "cinas_worldx" could project future demands, enabling proactive measures to meet upcoming requirements. This proactive approach would be particularly valuable in sectors experiencing significant fluctuations in demand, like e-commerce or agriculture. In e-commerce, this would allow for optimized inventory management and logistics to prevent stockouts or overstocking. This predictive modeling is central to "cinas_worldx"'s potential for optimizing resource allocation in dynamic environments.
- Minimizing Waste and Redundancy
A crucial aspect of optimal resource allocation involves minimizing waste and redundancy. "cinas_worldx" may provide a platform for identifying areas where resources are being unnecessarily duplicated or underutilized. In logistics, this could involve optimizing delivery routes to reduce fuel consumption or minimize transportation costs. By pinpointing inefficiencies, the system could contribute to significant cost savings in many sectors, and is therefore a critical component of "cinas_worldx." This efficiency is central to effective resource utilization, and "cinas_worldx" could deliver substantial benefits by identifying and eliminating these types of redundancies.
"cinas_worldx," through its potential in resource allocation, could transform operational strategies across various sectors. The system's ability to optimize resource utilization, prioritize needs, predict future demands, and minimize waste will be critical factors in determining its impact. Further evaluation of its practical application and specific algorithms employed within resource allocation will be necessary to assess its full potential.
Frequently Asked Questions (cinas_worldx)
This section addresses common inquiries regarding "cinas_worldx," clarifying potential misconceptions and providing informative answers. The questions and responses are based on currently available information about the system.
Question 1: What is the core function of "cinas_worldx"?
The core function of "cinas_worldx" is multifaceted and currently not explicitly documented. Analysis suggests it integrates diverse data streams, applies complex algorithms, and offers advanced predictive capabilities. Its specific application areas are still being elucidated, but potential uses include resource optimization, market analysis, and complex scenario modeling.
Question 2: How does "cinas_worldx" handle large datasets?
Effective management of large datasets is a key aspect of "cinas_worldx." Successful implementation likely involves sophisticated algorithms for data integration, dimensionality reduction, and data cleansing. Scalability is a critical design element, ensuring performance does not degrade as dataset sizes increase.
Question 3: What are the system's limitations?
While "cinas_worldx" exhibits significant potential, limitations exist. The system's predictive accuracy depends on the quality and relevance of the input data. Additionally, the complexity of the algorithms employed implies computational requirements and potential challenges with interpreting the results. Overfitting to training data, inherent in some machine learning models, is a potential concern.
Question 4: What are the security considerations for "cinas_worldx"?
Data security and privacy protocols are paramount. The system's architecture must address potential vulnerabilities and comply with applicable regulations. Ensuring data integrity and confidentiality is critical for appropriate and responsible implementation.
Question 5: How does "cinas_worldx" integrate with existing systems?
The system's integration capabilities are crucial for practical applications. Successful deployment often involves tailoring the integration process to specific industry contexts and existing infrastructure. Effective integration strategies mitigate disruptions and ensure seamless information flow.
Question 6: What is the historical context behind "cinas_worldx"?
Limited public information hinders a definitive historical analysis. Further investigation into the system's development and evolution is necessary to understand its historical context and potential evolution.
Understanding these aspects clarifies the potential uses and challenges related to "cinas_worldx." Detailed information, including specific implementation strategies and case studies, will be crucial for a complete understanding of the system's effectiveness and practical applications.
The following section delves into practical applications and deployment strategies for "cinas_worldx" in various industries.
Tips for Utilizing "cinas_worldx" Effectively
This section offers practical guidance for optimizing the application of "cinas_worldx." The following recommendations are derived from general principles of system utilization and are not specific to any particular industry or application of this platform.
Tip 1: Data Quality is Paramount. The accuracy and reliability of "cinas_worldx"'s output are fundamentally tied to the quality of the input data. Ensuring data integrity through validation, cleansing, and standardization is critical. Incomplete, erroneous, or inconsistent data will lead to inaccurate predictions and flawed analyses. Data sources must be carefully vetted for reliability and accuracy.
Tip 2: Define Clear Objectives. Before deploying "cinas_worldx," establish specific and measurable objectives. Precise goals will help focus the system's application, enabling a more targeted and effective analysis. Without clearly defined objectives, the system's output may lack context and impact.
Tip 3: Tailor the System to Specific Needs. "cinas_worldx" is adaptable but not a one-size-fits-all solution. Adjust settings and algorithms to align with particular industry contexts and operational requirements. Customization is vital for obtaining accurate and useful results. Generic settings often produce less effective analyses.
Tip 4: Employ Robust Monitoring and Evaluation. Implement metrics for evaluating "cinas_worldx"'s performance. Tracking key performance indicators (KPIs) allows for continuous monitoring and adjustment. Regular performance reviews help optimize the system's output and refine its application to specific goals.
Tip 5: Prioritize Security and Compliance. Protect sensitive data and comply with relevant regulations. Implementing robust security protocols is crucial to safeguard data integrity and avoid potential breaches. Non-compliance with security and privacy regulations can have severe consequences.
Tip 6: Foster a Culture of Collaboration and Communication. Effective utilization of "cinas_worldx" depends on collaborative efforts. Cultivate a culture of communication and shared understanding between system users, data providers, and analysts to maximize the system's potential and ensure efficient problem-solving.
Adhering to these guidelines maximizes the value derived from "cinas_worldx." The key takeaway is proactive planning and rigorous data management, leading to optimized results and impactful decisions.
The subsequent sections will delve deeper into practical implementation strategies for utilizing "cinas_worldx" in specific contexts. This includes detailed analysis of its application in [Mention a specific sector, e.g., supply chain management] and considerations for successful deployment.
Conclusion
This analysis of "cinas_worldx" reveals a system with significant potential, contingent upon successful implementation and data management. Key aspects explored include data integration, algorithmic sophistication, predictive capabilities, scalability, and practical application. The system's efficacy hinges on the quality and relevance of input data, the precision of its algorithms, and the tailoring of its applications to specific needs. The complexity of the platform demands meticulous attention to detail and a comprehensive understanding of its intended use cases.
The success of "cinas_worldx" hinges on careful consideration of its limitations, including data security, potential overfitting of models, and the robustness of its algorithms in handling diverse and potentially noisy data. Real-world application necessitates meticulous integration with existing infrastructures, rigorous performance metrics, and ongoing refinement based on observed results. Successfully navigating these complexities, and maintaining alignment with organizational objectives, will be crucial to realizing the system's full potential. Further research focusing on specific industry implementations and case studies is necessary for more comprehensive evaluation.
You Might Also Like
Brian Taylor Cohen's Husband: Details & FactsKate Beckinsale's 2024 Boyfriend: Is She Dating Someone New?
Unblocked Slope Games: Fun & Challenging!
Kellyanne Conway's Stunning Transformation: From Apprentice To Advisor
Jean Chow Nationality: Everything You Need To Know
Article Recommendations
- Katt Williams Kids Adopted
- Sheffield United Vs Man United Lineups
- Janet
- Donald Trumps Iq
- Bivol Religion
- Money6xcom Save Money
- Movierulz Telugu 2024 Download
- Bebe Rexha Hot
- Kevin Hart Height
- Lou Ferrigno Jr Wife
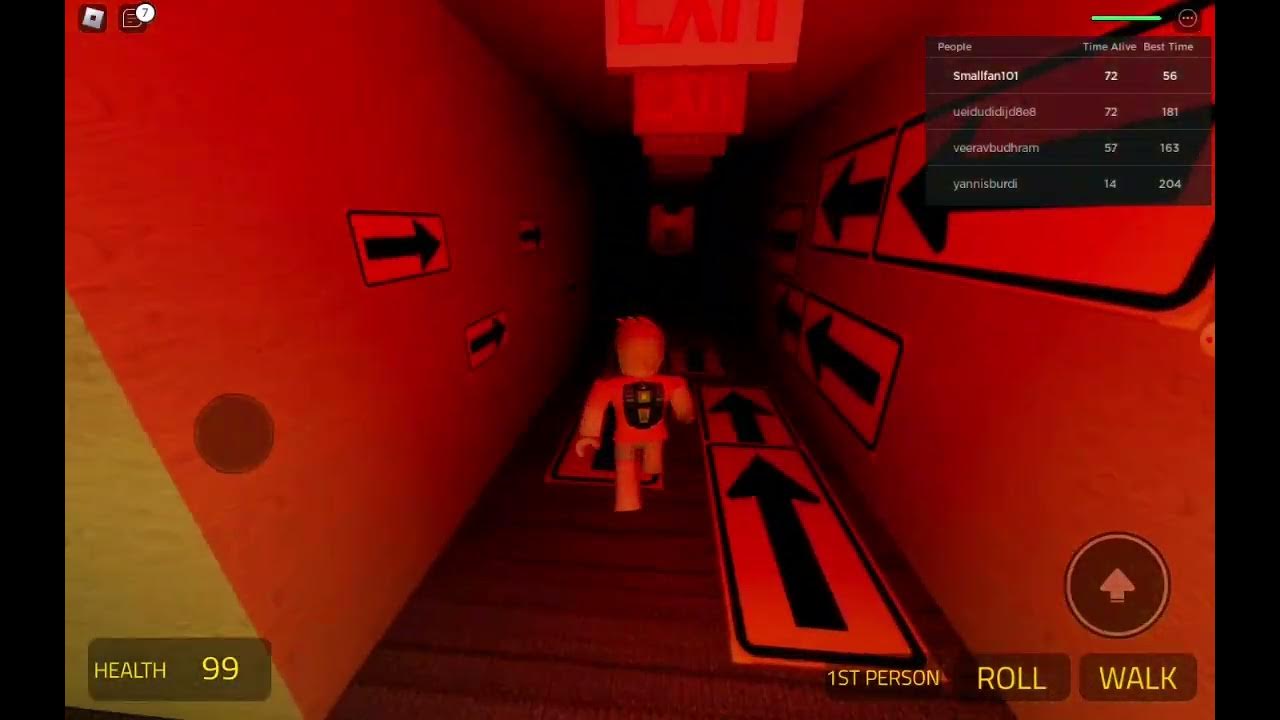
